We know that AI is poised to have an incredible impact on R&D in life sciences. But, as with any transformative change, moving from conception to ideation to reality means overcoming some very specific obstacles.
AI-driven R&D requires access to high-quality, well-organized data from diverse sources. It must be made findable, reusable, and machine-ready for modeling. However, overcoming data silos and integrating complex, multimodal datasets at an enterprise level is a nearly impossible task for most organizations. Many teams don’t fully trust their data. In a 2024 TDWI Best Practice Report, 48% of companies said that improving trust in data quality, consistency and completeness to move forward with their AI projects is their top goal.
In fact, life science R&D teams struggling to bring their AI initiatives to fruition often face a conundrum. Data intelligence alone is not enough. Scientific intelligence alone falls short. They need both: data intelligence + scientific intelligence. Together, Dotmatics and Databricks are making this a reality.
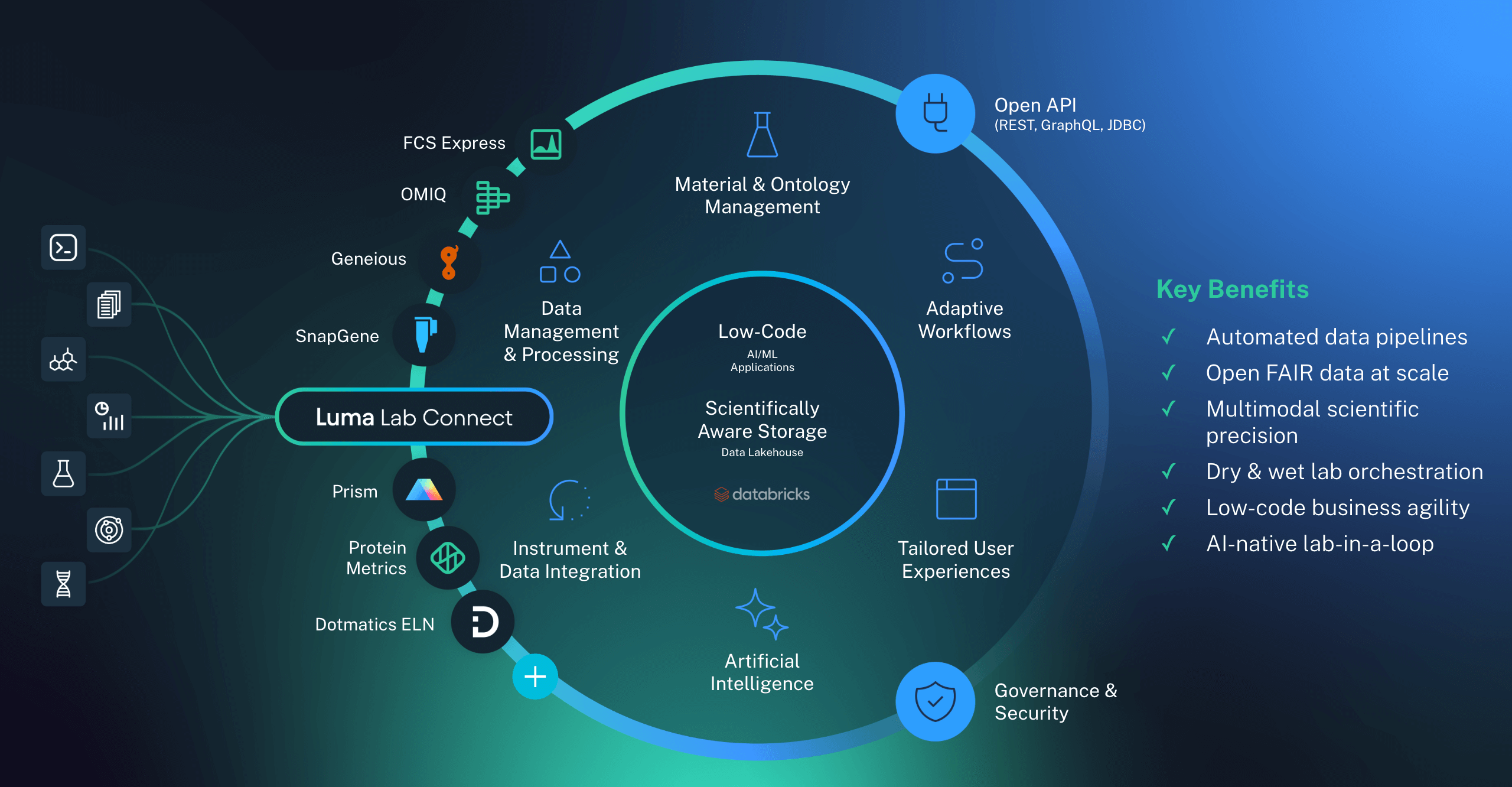
Luma leverages Dotmatics’ scientific technology, alongside Databricks’ data and AI technology, to enable R&D teams to build low-code scientific apps and create (and where possible automate) flexible workflows that connect science, data, and AI/ML in order to accelerate multimodal R&D.
Dotmatics & Databricks: Uniting to transform innovation
Last year, we announced a strategic partnership with Databricks. Dotmatics is one of the very first "built-on Databricks" partners, and our Dotmatics Luma is built on a platform of Databricks, leveraging a modern market-leading AI cloud that is optimally designed for scientific data.
Our aim is to catalyze life science organizations to drive deeper insights and accelerate discoveries, by providing them the ability to unify and analyze vast volumes of scientific data. Dotmatics Luma aggregates relevant scientific data into intelligent structures to support the entire Make-Test-Decide cycle across multiple modalities of therapeutic discovery. This end-to-end platform for discovery and development is supported by Dotmatics’ best-in-class scientific products including GraphPad Prism, Geneious, SnapGene, and Protein Metrics. Combined with Databricks’ ability to handle large, complex datasets, Luma can empower researchers in the life sciences with faster, more scalable pathways to deliver transformative therapeutics.
Recently, a panel of experts from Databricks, Dotmatics and TDWI research firm, shared insights on how the partnership is helping companies transform their scientific R&D data processes and support their advanced AI initiatives, unpacking four key themes:
What are scientists looking for in a modern data platform?
How can we empower scientists (and better utilize their time!) using AI and automation?
What are some exciting ways LLMs and AI generally are transforming scientific discovery?
What non-tech strategies should you consider to improve collaboration and outcomes?
Scientists want a modern data platform
Any organization hoping to leverage the power of AI needs a modern data platform that supports collaboration across teams, ensures robust security and governance, and provides easy access to data and AI algorithms. Essential characteristics include:
Open and FAIR
In life sciences, data management is at the heart of successful research, and the FAIR principles—making data Findable, Accessible, Interoperable, and Reusable—serve as a guiding framework. Databricks, through its Unity Catalog, is helping organizations adhere to these principles. Unity Catalog offers governance for not just data, but also model assets with built in search and discovery. To ensure proper access, that’s combined with attribute-based access controls, where an individuals’ role or team can be tied to levels of access for a particular data or model. Open data standards like Delta Lake and Iceberg are also supported, making it easier for teams to share data across platforms and tools, and ensuring data is interoperable.
Scalability
As data in life sciences grows increasingly complex, having a platform that can handle diverse data sources is crucial. Databricks' scalability is key, especially for industries like biologics discovery, where terabytes of data are generated. For example, one customer using Dotmatics Luma Lab Connect pared 42 billion data records last year alone, solely originating from instrument data. When you factor in all the other sources of data that exist in the dry lab and wet lab, it becomes essential to bring them together in a way that enables those data sets to be correlated and represented with a high degree of scientific precision.
Scientific smarts
Dotmatics enhances Databricks’ capabilities with “scientific smarts,” such as a chemistry and biology engine, providing the ability to represent sequences and chemical structures within the Databricks ecosystem. Luma not only stores and processes large datasets, but also integrates with industry-leading scientific applications like GeneiousPrime, GraphPad Prism, and Protein Metrics, providing the scientific context needed to analyze the data effectively. Data context and lineage are essential for understanding how data evolves, from molecule design to testing; a good platform ensures that data is connected and contextualized, making it easier for researchers to make informed decisions.
ML / AI interoperability
One of the reasons Dotmatics chose to build on top of the Databricks platform is its ability to natively interoperate with machine learning (ML) tools. That includes the ability to store models, store training and training weights inside of Unity Catalog and allow governed access to those models. Advanced functionality like predicting chemical activity structures to predicting an antibody or protein structure liabilities, or whether a protein will fold correctly or not, depends on these models.
Empowering scientists with AI and automation
Data preparation is often the unsung hero of data science and scientific discovery—a crucial yet time-consuming step that consumes significant bandwidth. We know that 50% of scientists and data scientists' time is actually spent on data extraction and transformation. They're also five times more likely to repeat experiments due to data issues. TDWI reports that of the top priorities for improving data management and governance, the biggest three are reducing time and cost associated with data collection and preparation (43%), increasing data availability for model development (40%), training and testing, and making it easier for users to operationalize data pipelines and transformation (32%).
Databricks and Dotmatics are addressing this challenge head-on, leveraging innovative technologies to streamline workflows and empower users to focus on meaningful, value-driven work. We think that scientists should be able to jump straight into their most impactful data science projects. That’s why Databricks has integrated foundational capabilities into its platform, such as built-in quality controls and the innovative Lakehouse Monitoring system—an AI-driven tool for anomaly detection—which allow for seamless orchestration across pipelines. By automatically identifying and quarantining issues, these tools enhance data integrity and free up users to attend to higher-value activities.
While Databricks focuses on the data science, Dotmatics focuses on the immensely challenging domain of scientific workflows, blending the creative and structured aspects of research. Science isn’t about rigid workflows; science requires flexibility. Dotmatics’ Luma platform offers adaptive workflows where tasks are validated by their inputs and outputs, not by a pre-defined order. This approach empowers scientists to execute tasks as they see fit while ensuring data is captured and organized automatically.
With Luma, data relevant to each workflow step is linked and tracked without manual intervention. This captures both dry and wet lab processes organically, easing downstream data wrangling and allowing researchers to focus on discovery. Data automation is equally tricky, because data automation without a unified platform ultimately leads to silos and fragmentation. It's much harder to wrangle across 50 systems than it is to wrangle within one. Co-locating data simplifies wrangling and provides a scalable foundation for workflows.
Leveraging AI to transform scientific innovation
AI is transforming how organizations approach data and drug discovery, and it's incumbent upon technology providers to build AI into platforms that accomplish, automate and improve the quality of a number of tasks, for example:
Databricks is building AI into its Unity Catalog. That means that behind the scenes, large language models (LLMs) auto-describe tables and columns, simplifying workflows for users so they can focus on the more exciting aspects of discovery.
LLMs can combine vast amounts of data. Imagine using AI to harmonize medical literature with internal research data, plus all of the instrument data Dotmatics is able to bring to bear—that creates a really powerful opportunity to build AI powered assistants. Or consider the possibilities of using AI with pre-training models, such as geneformer models to better understand gene expression and network biology. LLMs trained on biological data are now being used to generate proteins or antibodies based on specific characteristics. While we’re still in the early days, its potential is enormous.
Retrieval-augmented generation is an exciting development. Retrieval-augmented generation allows AI to find relevant data with simple text queries. Imagine scenarios where teams are looking for the needle in a haystack and want to be able to search through reams of data with just an open text box. By vectorizing data and comparing it to questions, organizations can find exactly what they need quickly. Dotmatics is implementing this functionality in Luma, thanks to native platform support offered by Databricks.
Non-generative predictive ML still plays a crucial role. This is especially true in the lab. It helps predict which molecules will be effective, saving time and resources in the discovery process. And generally thanks to AI, scientists should expect to experience a number of small improvements in routine tasks without ever even knowing the AI is present.
Beyond tech: Enhancing collaboration through better strategies
Successful collaboration isn't just about technology—it's about people, processes, and aligning on the right business outcomes. You want to create a framework that allows for opinions to be heard and trade-offs to be evaluated. Before diving into tools, it’s critical to first understand: what are the business objectives and outcomes that the collaboration is trying to achieve? Once everyone is clear on the goals, technology can facilitate the necessary debates and decision-making around the data and trade-offs.
Control or security governance is a common challenge that organizations lack when sharing data. This often means that collaborators must learn custom APIs or use tools they’re not comfortable with, hindering effective data analysis. For collaboration to thrive, teams need to be able to use their preferred tools—whether it's a BI tool or Python. A governance system like Unity Catalog lets organizations securely share data while maintaining flexibility, giving teams confidence to work with data in their chosen tools, which is key to kickstarting successful collaboration.
Low-code technology is a key driver of successful collaboration. It’s not just about simplifying software development; it’s about democratizing the process and bringing key stakeholders from different functions to the same table. The goal is to allow business, science and IT stakeholders to actively participate in building software, so that they can quickly see whether it meets their needs before committing to months of development. This approach accelerates agility and ensures the project is on track to meet user requirements.
Data management plays a crucial role. A low-code platform, particularly one built on technologies like Databricks, centralizes data and offers visibility across various sources. With AI-powered, configurable interfaces, everyone can interpret data through a consistent lens, preventing different functions from interpreting the same data set differently. This alignment helps foster better collaboration.
Change management is also key. Researchers and teams are used to their workflows, and shifting those habits can be challenging. It’s important to slow down, focus on collaboration, and recognize that a bit of delay upfront can lead to faster progress in the long run. As research boundaries evolve, particularly in fields like drug discovery, enabling seamless collaboration is more important than ever.
On-demand webinar: Building breakthroughs - harnessing data and AI for innovation
If you’re interested in learning more, check out the full webinar, Building Breakthroughs - Harnessing Data and AI for Innovation. Hear our panel of experts dive deeper into the ways in which the Dotmatics and Databricks partnership is helping R&D teams optimize their:
Data acquisition to consistently and securely collect data and metadata at scale
Data infrastructure to better organize and manage multimodal data and metadata
Data readiness to reduce the burden of precursory AI data preparation processes
Data integration to enable advanced analysis of complex heterogeneous data with speciality scientific tools and multimodal AI/ML algorithms
Data governance to safeguard and track data use across teams and within AI models
Panelists include:
Kalim Saliba, Dotmatics, Chief Product Officer
Michael Sanky, Databricks, VP, Healthcare & Life Sciences GTM
Scott Stunkel, Dotmatics, VP, Engineering - Luma
David Stodder, The Data Warehousing Institute (TDWI), Research Fellow