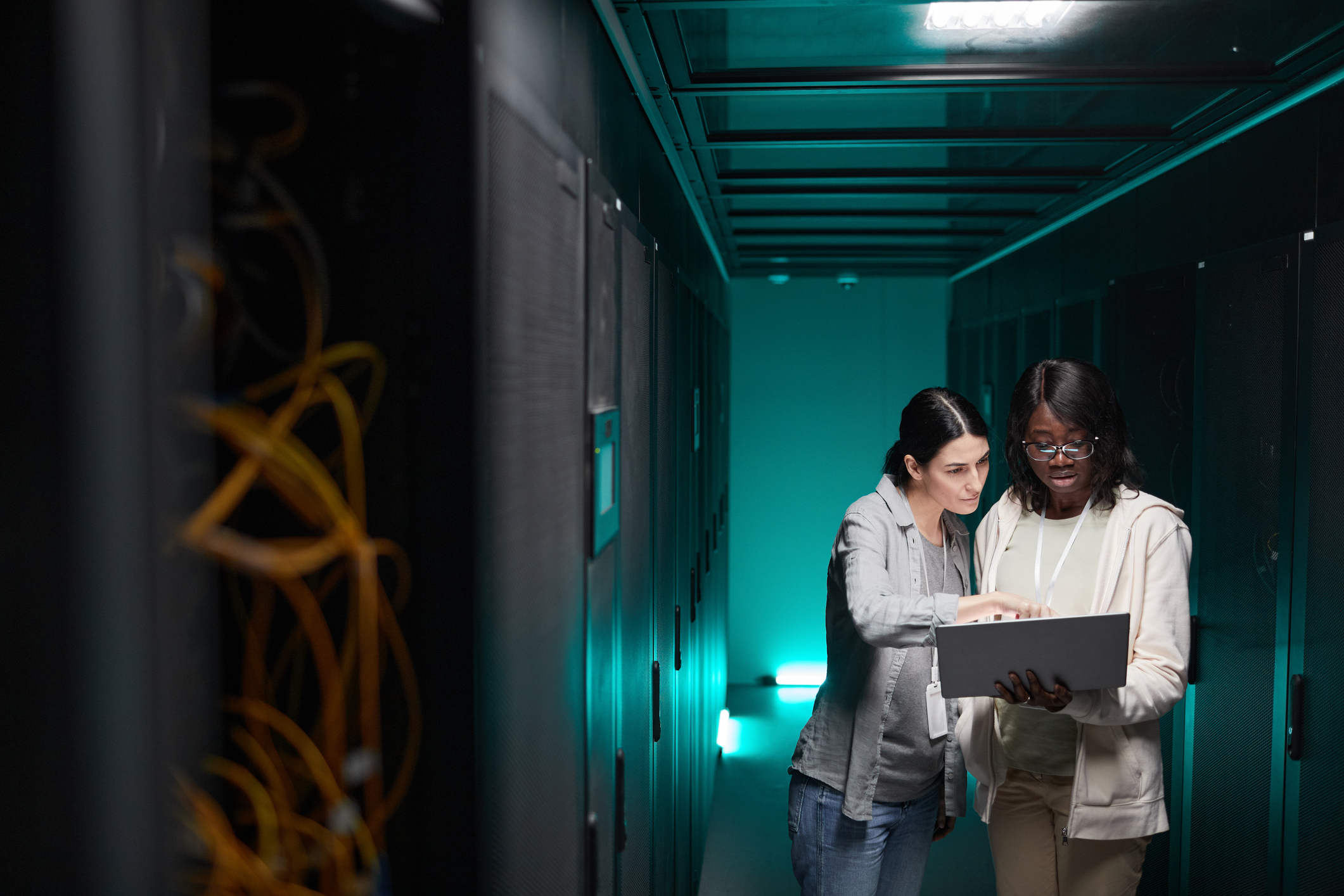
The Dawn of AI in Drug Discovery
ChatGPT and other generative large language models (LLMs) are becoming increasingly pervasive in our personal and professional lives. In the life sciences, for example, the use of AI is nothing new, but it is certainly growing. In fact, McKinsey reports that, “The AI-driven drug discovery industry has grown significantly over the past decade, fueled by new entrants in the market, significant capital investment, and technology maturation.”
As the use of LLMs grows, it has become clear that any potential benefits come along with numerous challenges. We must consider factors such as:
Data quality and transparency – Is the quality of data going into, and coming out of, generative models sufficient for its intended purpose?
Truth dilution – How can models and algorithms avoid perpetuating quality issues and diluting the truth?
Complexity management and training sufficiency – Have the models been properly trained using accurate and sufficient data? Are the questions being asked too complex or specific for general algorithms that have been built with broad training datasets? Will results be unreliable or in need of expert scrutiny?
Below, we explore some broad examples to illuminate key considerations that must also be kept in mind as we increase our adoption of AI in scientific R&D and integrate it into our primary workflows.
From the text-prompt-to-image app Craiyon to the photo-remixing tool Midjourney, AI-based apps have become increasingly popular. Growing use of such apps feeds developers more and more training data, however there is generally insufficient assessment on whether such data are inaccurate or proprietary, as evidenced by disputes over Midjourney’s use of output images that had artists’ signatures visible. Similarly, in scientific research, data origin is of key importance as results quality and ethical collection must be ensured.
A fun example to illustrate context concerns, specifically in using LLMs, is mixology, which in many ways is analogous to product formulations. A prominent YouTube mixologist used ChatGPT to create cocktail recipes from a preset list of ingredients. Not surprisingly, some results were unpalatable because crafting a cocktail recipe isn’t just a matter of following a defined format, but rather an art that relies heavily upon contextual application of both knowledge and sensory inputs. The mixologist’s assessment was that ChatGPT might best be used as an assistive tool, not a primary recipe generator. The role of LLMs in research must be similarly augmentative, helping to fuel scientists’ creativity, not replace it.
AI-written articles have become more prominent than most of us realize and are a great example to illustrate data accuracy challenges. Earlier this year, Buzzfeed News reported that technology news outlet CNET had generated 70+ articles using AI, without prominently disclosing such initially. As a follow-on, Buzzfeed then used ChatGPT to generate their own article on the matter, noting that the process was error-prone and they had to rewrite their prompt several times to avoid basic factual errors. In the scientific realm, teams go to great lengths to ensure their data are trustworthy. Increased use of chat-based AI will present new challenges for doing such.
Lexical analysis, or natural language processing (NLP), has been around for years. For example, there are a number of solutions for scanning papers and building semantic models. In drug discovery, researchers might use such tools to scan publications to quickly uncover potential binding targets for small molecules. While these tools can help parse through large volumes of content in rapid fashion, they’re certainly not fool-proof and manual consideration is often necessary to make final assessments. This is partly due to the inherent challenges of conveying complex information in written publications. What constitutes a “good” paper is a discussion far beyond the scope of this piece; but, certainly, most of us have read papers that left us wondering if we were missing some assumed knowledge, or if the paper was just poorly written. Training models using such papers is bound to be challenging.
Complicating matters even more is the growing popularity of using AI algorithms to generate new content using source materials of varying quality. The output content may often sound factually correct even when it isn’t, or it may become too complex and confusing to interpret. This can amplify quality issues and will likely skew toward poor-quality; in turn, readers may feel like they actually need algorithms to interpret information; but if those algorithms are themselves lacking, the quality problem just self-perpetuates, further diluting the content and making the truth increasingly difficult to decipher.
ChatGPT is also being hailed for its ability to write code; but like written language, creating code is an artform in its own right, and the more complex the code is, the greater the chance of error. Say, for example, you ask for the creation of an “alignment algorithm” without further specification. You may be given an algorithm that can align peptide sequences, but not DNA sequences. Because the letters representing DNA bases—A, C, G, and T—are also used to denote amino acids, you might get an output without error, but it might not actually be what you’re looking for. This leaves highly skilled people to clean up after the algorithm. Their skills, which have been acquired through years of computational life sciences work, might be better applied to actually write and refine the algorithms themselves.
As this example above illustrates, lack of specificity is a fundamental obstacle that must be kept in mind when employing any AI tools. Generalized models that have been trained on huge datasets with no specificity will undoubtedly struggle in specialist areas. For example, in drug discovery, if a predictive algorithm has been trained on small molecules for protein-drug binding, the trustworthiness of its binding predictions depends on how structurally similar the input molecules are to the molecules in the training set. In such cases, an uncertainty metric can help improve transparency, letting users know the limitations of the model. This notion of trustworthiness is of key importance. Models, after all, are only as good as the quality of their training data. Without transparency, how are we to know if models were trained using insufficient, inaccurate, or improperly sourced data? While definite, confident answers like those given by ChatGPT may be attractive, those answers mean little without a trustworthiness score or insight into training-data sourcing and quality.
Ask any scientist and they’ll likely agree that the use of machine learning and artificial intelligence in R&D is nothing new. For more than a decade, researchers have used computational techniques for many purposes, such as finding hits, predicting binding sites, modeling drug-protein interactions, and predicting reaction rates. Most scientists will also likely agree that all models, like all data, aren’t created equal. In many cases, AI -and ML-based tools have largely been used supplementally, not exclusively, but as they become more of a mainstay in our standard workflows, we must keep in mind the concerns illuminated by our examples above.
Developers of AI tools should aim to build semantic relationships into neatly organized training data and provide interpretable metrics that allow users to gauge confidence and reliability; users should not be expected to blindly take predictions at face value. It’s akin to providing a satellite navigation system that empowers drivers to see where they are and identify the best route to get where they need to be, rather than forcing upon them a self-driving vehicle that requires them to relinquish all knowledge and control. It’s about using AI to augment people’s expertise, not replace it (or them).
It all boils down to this: AI holds incredible potential to help speed up work, save costs, inspire innovations and expand the scope of possibility, but undoubtedly, the necessity of clean data, trustworthy models, and human insight is still imperative.
The Dotmatics Platform facilitates easy capture of clean data and enables the integration of AI into more extensive R&D workflows.
Request a demonstration of Dotmatics to learn how we can help you get AI-ready.