What’s Complicating Good Data Practices and Data Integrity?
Introduction: An Evolving Pharma Landscape
The evolving pharma landscape is affecting not only the drugs coming to market, but also the ways researchers work in the face of changing technologies and growing pressures. Large-molecule biologics have gained ground on small-molecule, chemically-based drugs that historically dominated the market. Today’s market and pipeline have grown increasingly diverse and now encompass a broad range of modalities, such as antibodies, proteins and peptides, cell therapies, gene therapies, nucleic acids, small molecules, and chemically-modified and conjugate therapies.
At many companies, single modes of discovery have given way to multimodal approaches for developing therapies. Scientists are addressing targets via whatever manner is best in an attempt to open up hard-to-reach target space and develop novel treatments, as well as stave off growing business pressure from rising costs, high failure rates, and looming patent cliffs. While this multimodal approach to R&D is promising, it is also difficult to enable within environments that have been domain-segmented for decades. Multimodal R&D data are incredibly diverse and not inherently compatible, much like the technology used to produce and analyze that data; these incompatibilities impede workflows, complicate collaboration, force experts to waste time on integration and data tasks, and obscure connections buried amongst datasets.
The impact of this data and technology disconnect will only become more severe as R&D teams move into an AI-assisted future, in which they aim to leverage scores of diverse public and proprietary data to help focus R&D efforts and reduce timelines and costs. As organizations adopt multimodal approaches to discover therapies, they need technology partners who can support diverse modalities of R&D that produce multiple unique data streams.
The Spread of Multimodal Data in Pharma
Technology advances in both research and patient care have created a deluge of data, including instrument and experimental data, clinical study results, structure and sequence data, omics data, patient data, patent data, publication data, etc. Making connections between these different datasets is essential to developing impactful medicines and driving more personalized patient care. Unfortunately, all of this data is often trapped in different formats and systems, making it difficult to integrate and share—a challenge made more glaring in the face of data-sharing initiatives, such as the FAIR Guiding Principles for scientific data management and the National Institutes for Health (NIH) Data Management and Sharing Policy.
Benefits of Multimodal R&D
In order to fully leverage multimodal R&D data, organizations must digitally transform their R&D environments. They need to modernize the ways they collect, collate, format, and model data, with the ultimate goal of amassing and correlating high-dimensional target, disease, and drug data that will help guide R&D efforts. The potential benefits of multimodal R&D include:
Enhanced Drug Discovery: With multimodal R&D, researchers can interrogate the target space from multiple angles in order to better understand the disease state and uncover and develop novel therapies of any type.
Accelerated Timelines: Well curated multimodal R&D data can be used to inform predictive and generative models that reduce development cycles and shorten time to market, such as those that identify and validate targets, screen or suggest compounds, and identify biomarkers. (Hear Dotmatics’ Vice President and Global Head of Science and Technology, Alistar Campell, talk more about this topic with DocWireNews.)
Improved Trial Design: Multimodal data, including small molecule descriptors, AMDE-Tox data, transcriptomic data, text-based drug and disease representations, clinical trial protocols, publications, and patent data, can be holistically considered to help optimize trial endpoint definitions, stratify patient subgroups, and estimate treatment effects.
Challenges of Managing Multimodal Data
Successful digital transformation in a multimodal R&D environment is an art of connecting science, data, and decision making. While challenging, it is essential to driving efficiencies, improving collaborative decision making, and powering predictive and generative solutions. Key hurdles organizations must overcome when managing multimodal R&D data include:
Data Volume and Complexity: Multimodal R&D produces huge volumes of diverse data, including structured, semi-structured and unstructured data, sequence data, chemical structures, numeric data, text, images, metadata. All of this complex data must be properly processed and stored; if it is not easily findable, accessible, or (re)usable, its research value plummets.
Interoperability and Integration: Multimodal R&D data flows in from a wide range of lab instruments, equipment, and systems, making its collation a technical and administrative challenge; these data producers are generally not inherently compatible or easily integrable, and they typically output data in different, often proprietary, formats, making that data difficult to model and correlate.
Data Quality and Governance: Ensuring data accuracy, consistency, and integrity can be difficult in multimodal R&D, where cross-functional teams are working with different specialty tools and workflows. Time-consuming and error-prone movement of data between different systems can be avoided with a connected R&D cloud platform that centralizes and standardizes diverse data at scale, readies it for downstream use, and provides tools for data management and governance.
Dotmatics Luma Multimodal Discovery Platform
As pharma and biotech companies move from single modes of discovery toward a multimodal, AI-assisted future, they need an R&D platform that can evolve with them. Dotmatics Luma™ is a breakthrough multimodal discovery platform that simplifies the collection and processing of multimodal R&D data and helps non-technical users make correlations and gain critical insights. Key elements of Luma include:
Instrument Integration: Connect to virtually any data source (e.g., scientific applications, ELNs, instruments, libraries, registries, files, CRO uploads) to pull in data of any volume, type, or format (e.g., structured/semi-structured/unstructured data, sequence, numeric, text, image, metadata, etc.).
Data Modeling: Centralize and standardize on a common, cloud-based, data-processing platform that can handle data at scale and ready it for downstream use.
Advanced Analytics: Empower users to find, share, and output harmonized data into workflows, specialty apps, analytics and modeling programs, AI/ML algorithms, etc. so that they can garner the insights that will help them optimize R&D efforts from the earliest days of discovery and onward.
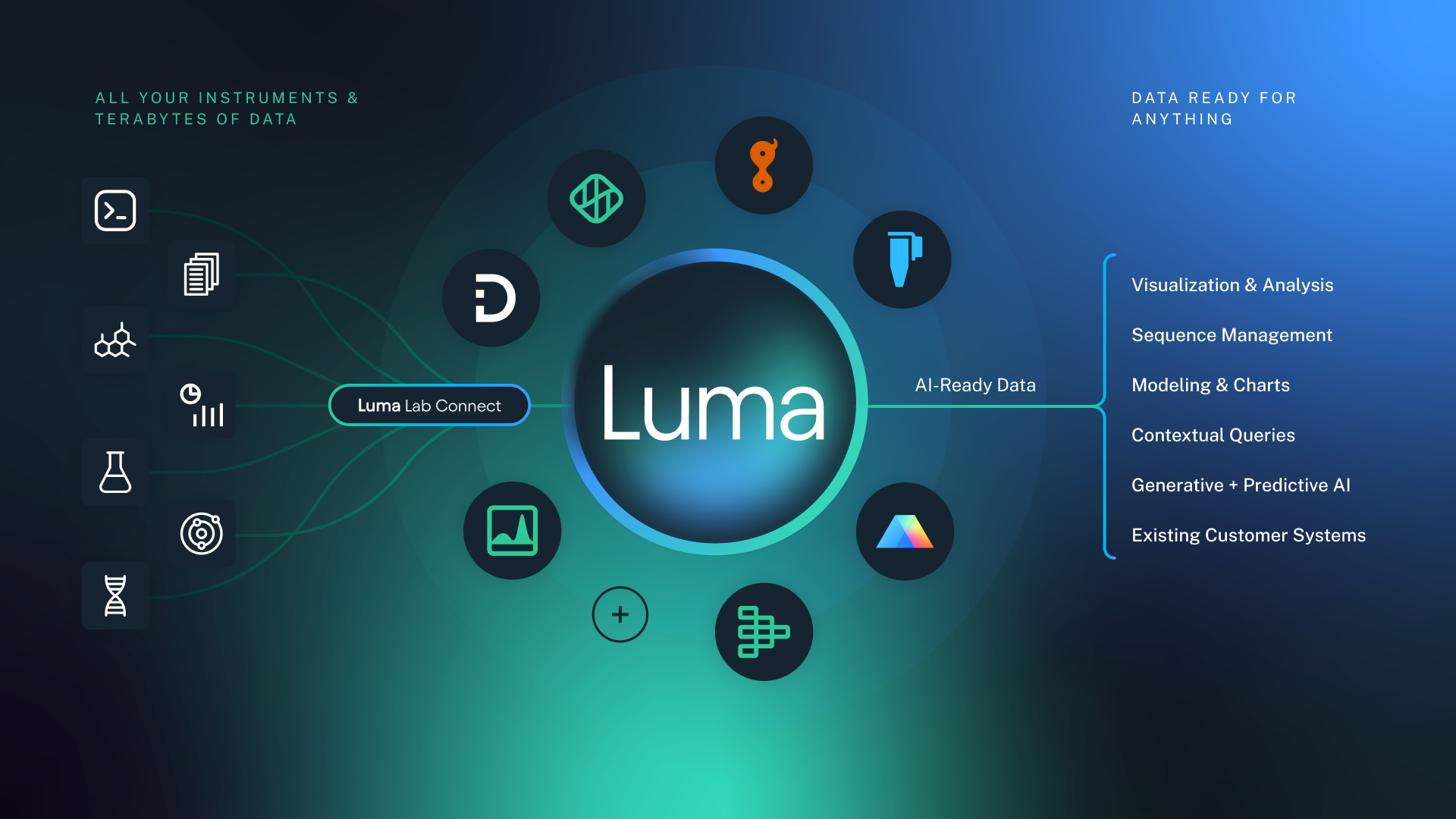
Learn more about the Dotmatics Luma multimodal scientific R&D platform and its Luma Lab Connect lab-integration solution.