Part 1: The Challenges of Selecting the Right Scientific Informatics Platform
Discovering materials or biological agents with novel properties and capabilities requires extensive research and development. The term “platform” is used frequently in the discussion of software used to facilitate these new discoveries. However, while the term is used frequently, it is highly ambiguous. In fact, in many cases, it is used as shorthand to plug conceptual gaps instead of explicating required functionality in detail.
“Platform” is a broad term. If something is a platform, it must cover a lot of bases, right? In many cases, it is in fact the opposite because of the potential vagueness of the term papers over a lot of gaps. In the last twenty years, there has been a dramatic deepening in scientific understanding and a corresponding increase in the kinds of modalities of treatment open to investigation (RNA-based therapies, cell therapies, more targets for vaccines, etc.). There has been a corresponding revolution in basic IT technologies available to create user-friendly, scalable solutions. The vendor and IT support community is struggling to harness the power of these capabilities to get better software into the hands of scientists more commensurate to the needs of today’s scientific context.
Key Requirements for a Scientific Informatics Platform
The potential scope of a scientific informatics platform is illustrated in the diagram below. The black rectangles indicate scientific activities with the implication that a platform includes the detailed workflow support required to perform the activity. The blue software package symbols represent the software components required to support both these scientific workflows and the data flows between key components. Together, this becomes the definition of a comprehensive scientific informatics platform.
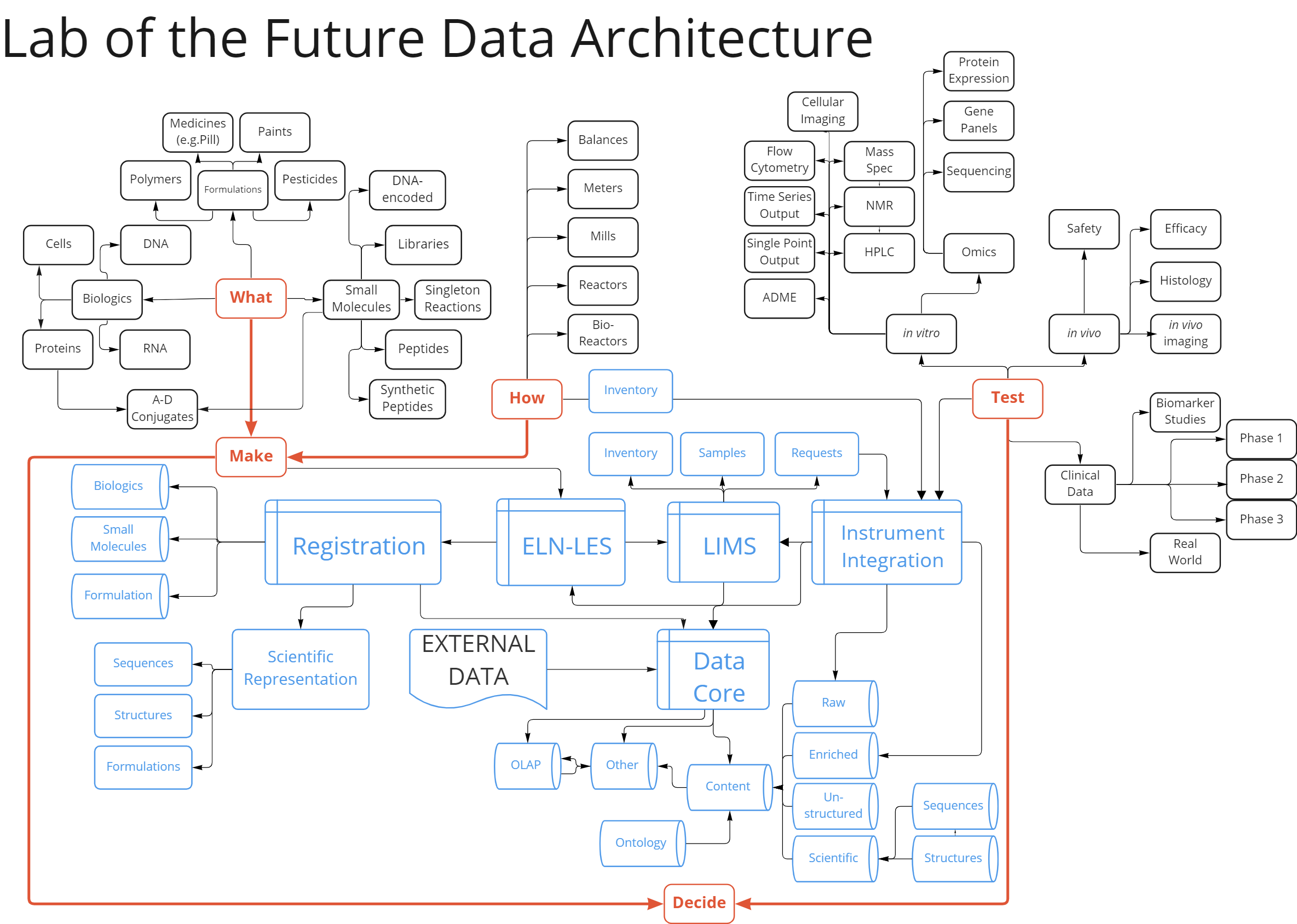
Figure 1: Platform Scope – the required scope of IT platforms has increased – has IT kept up?
Appropriately, a key requirement of a science informatics platform is to make workflow more efficient. Science is intensively iterative and collaborative. Things are made as potential products, to facilitate the discovery of products, and then to optimize how these new discoveries are brought to market efficiently and safely. The results of each iteration are evaluated in context to decide what to do next. The faster this cycle of innovation goes, the faster new discoveries are made.
As software facilitates individual workflow tasks, it opens the possibility for data to be structured and ingested into decision support systems. Workflow support potentially facilitates data flow support. Research experiments are recorded in the ELN component. As projects progress into development, the role of the ELN is supplanted by the Lab Execution System, which tracks individual process steps. Materials are identified in the registration component. LIMS is used to track the testing of new materials. Instrument integration capabilities streamline the flow of data from scientific instrumentation. Ultimately, all the data produced needs to go into a comprehensive decision support system.
It sounds simpler than it is of course. The scope of these requirements is large given the sheer complexity of the undertaking, and the underlying science gets ever more complex every day as our appreciation for the intricacies of nature grows. Each object in the above diagram is in fact its own universe of particular requirements.
The testing component of the innovation cycle is particularly challenging given that advances in instrumentation and analysis software are at the forefront of scientific advance, which means that ever more powerful and unique software is required to analyze the data sets coming off the new instruments. For example, our understanding of just the science around the mass spectrometry of proteins has improved immeasurably in the last several years with corresponding improvements in software available to resolve protein MS. How much capability can exist in a platform?
Meanwhile, organizational complexity adds another dimension of requirements. Different groups in large organizations will frequently chart their own course, and in many cases, critical data is produced externally. Interoperability between the platform and functions external to the sponsor organization is essential, and in many cases, interoperability between platform components and third-party systems is generally viewed as equally essential. Given the constraints, organizations investigating the implementation of a scientific platform have an important question to ask: is a scientific informatics platform even feasible given these functional and organizational challenges?
In my next post on this topic, I discuss effective data management given the inherent diversity and scale of scientific data. View this article now.