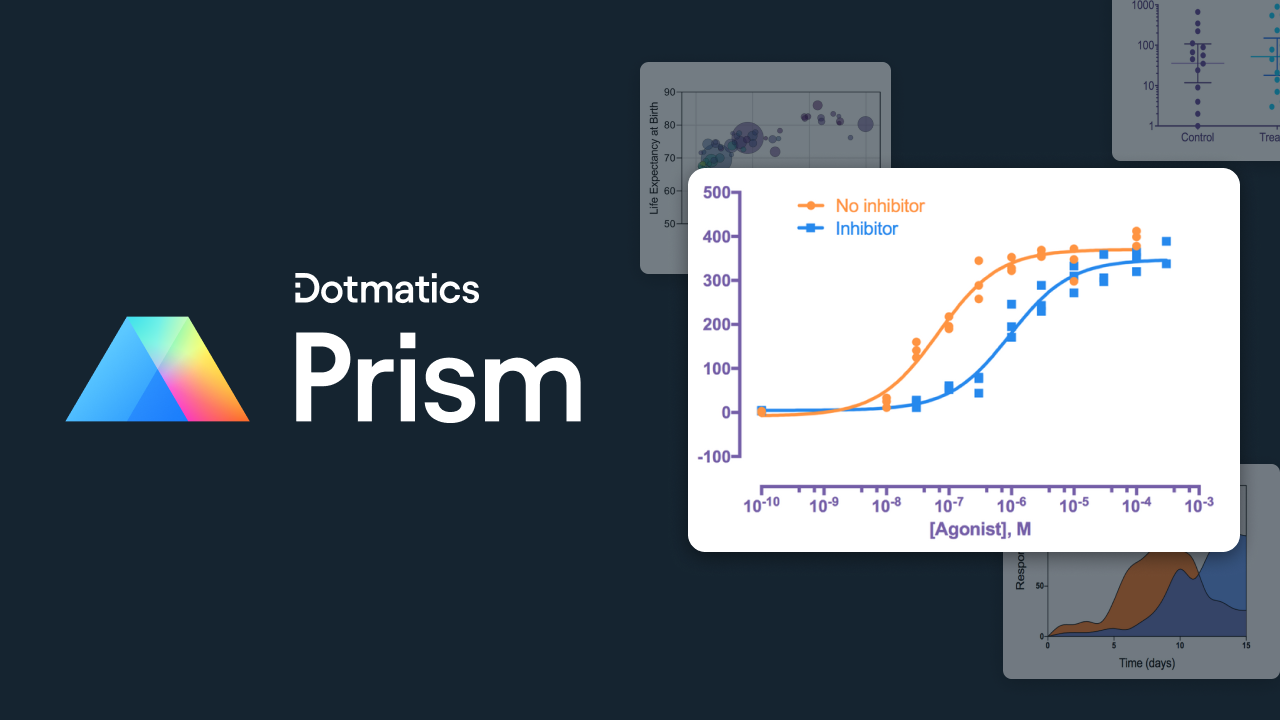
Embracing FAIR Data Principles with GraphPad Prism
Over the past decade, data sharing in scientific R&D has evolved from an anomaly to a necessity. Ten years ago, The Atlantic published an article titled, “Scientists Have a Sharing Problem,” which highlighted how competition, disorganization, and lack of standardization were impeding data sharing and scientific progress.[1] Fast-forward to today and most researchers now face a slew of data-sharing policies to which they must adhere. The impetus behind such policies ranges from fulfilling funding and publication requirements, to helping increase research citations, to encouraging sharing for improved collaboration and the greater good of science.
One notable policy is the National Institutes for Health’s (NIH) Data Management and Sharing Policy, which went into effect in 2023.[2] The policy lays out the ways in which research funded by the NIH must be made accessible to others through the use of detailed data-management plans and public sharing of both data and metadata using common standards. The inclusion of metadata in the NIH’s policy is illustrative of a persistent concern within scientific R&D—research reproducibility. Sharing data about the specific experimental conditions, collection methods, and analysis procedures used to generate data helps ensure the work can not only be tested and validated, but also reused and built upon to spark further innovation. The NIH’s data-sharing policy is just one of many spreading across the global scientific R&D landscape, from agencies in Europe and China, to the World Health Organization, to the U.S. White House Office of Science and Technology Policy (OSTP).[3-6]
Beyond a mindset shift toward data sharing, a technology shift is also needed. Researchers need to be empowered to more easily share data, but the complexity of the modern lab likewise complicates data sharing. While some disciplines, like genomics or crystallography, have made great progress embracing sharing, others are farther behind, in part due to challenges such as complex, non-standardized data types and a lack of common standards, ontologies, repositories, or platforms. On top of this, sharing is further complicated because researchers today are more often remotely collaborating across disciplines and departments; they are using a wide variety of technologies that are resulting in skyrocketing data volumes, diverse data types, and disconnected data sources. Often, specialty software keeps key data and metadata obscured by proprietary formats. As a result, cumbersome curation and preparation processes may be needed to ready data for sharing. And once data is prepared, facilitating safe and secure sharing of huge volumes of data presents yet another obstacle.
Challenges like these were a key driving force behind initiatives like the FAIR Guiding Principles for scientific data management, which were established in 2016 to promote the adoption of technology and processes that make all data findable, accessible, interoperable, and reusable by both humans and machines alike.[7] The FAIR Principles can help define requirements to which solution providers should adhere in order to ensure their solutions empower researchers to more easily share and reuse data.
How does using software, tools, or infrastructure built in the vision of FAIR help R&D teams?
Better Reproducibility - Support transparency, credibility, and research reproducibility by enabling detailed recording of the methods used for data collection and analysis.
Reduced Time and Costs - Avoid manual, inefficient, and error-prone data handling by automating processes where possible and facilitating better dataflow between research groups and the technology they use to innovate.
Faster Insights - Enhance your team’s ability to build off collective knowledge from public and proprietary sources, and prepare their data for advanced analysis, including with AI and ML.
In a survey conducted by researchers from the University of Manchester, pharmaceutical R&D teams who were implementing FAIR data principles noted benefits such as reusability of data (particularly legacy data that could be leveraged in AI-based analysis such as drug repurposing initiatives), as well as cost savings, such as through avoiding redundant experimentation and more easily finding existing datasets across previously fragmented departments.[8] In a broader assessment of the impact of FAIR across industries, an analysis by PricewaterhouseCoopers on behalf of the European Commission estimated that a lack of FAIR research data costs the European economy at least €10.2bn annually.[9]
A natural follow-on concern in the face of wide-spread data-sharing initiatives is data protection. One important distinction to make is that FAIR doesn't necessarily mean open. Data and tools can at once be FAIR compliant, while still safeguarded with controlled access to sensitive data. Data is accessible if a machine can automatically understand its parameters, but that doesn't mean all data needs to be freely available. This has many organizations questioning how they can support data sharing, while still properly controlling data access and ensuring security and IP protection. What measures should be put in place to ensure privacy and access control? What is the safest way to store and transfer data in the age of sharing? Will the controls in place persist across the different databases, tools, and systems teams use? These are likewise priorities for us at Dotmatics as we built solutions that not only help R&D teams share their data more efficiently, but also uphold stringent standards for data security and IP protection across an ecosystem of software, tools, instruments, and systems.
At Dotmatics, we’re building an ecosystem of R&D solutions that are aligned with FAIR principles and support collaborative, data-driven innovation. We help teams eliminate slow, error-prone, unFAIR data practices that can hinder research productivity, obscure research transparency, impact reproducibility, and ultimately compromise data integrity and security. We do this by prioritizing both:
Workflow - We are optimizing our UX/UI to help users easily find, access, share, and use data within and between products, such as our ELN and data discovery platform and our scientific specialty tools like Geneious Prime, Geneious Biologics, GraphPad Prism, and OMIQ.
Dataflow - Behind-the-scenes, data is ingested, modeled, stored, processed, and transferred in a manner that supports (re)usability, integrity, and security across an ecosystem of Dotmatics, third-party, and user-built solutions.
A great example of workflow and dataflow coming together is the recent release of our leading analysis and graphing solution for scientific research, GraphPad Prism, which now features a new, more open, FAIR-aligned file-format.
See for yourself the difference that a focus on whole-ecosystem workflow and dataflow can make in data sharing and collaboration. Contact us for a demo.
Puniewska, M. Scientists Have a Sharing Problem. The Atlantic. December 15, 2014. https://www.theatlantic.com/health/archive/2014/12/scientists-have-a-sharing-problem/383061/
Final NIH Policy for Data Management and Sharing. Notice Number: NOT-OD-21-013. https://grants.nih.gov/grants/guide/notice-files/NOT-OD-21-013.html (Accessed 2/22/2024)
Kaiser J. and Brainard, J. Ready, Set, Share! Science, Vol 379, Issue 6630. January 23, 2023.
A European Strategy for data. European Commission. Website. (Accessed 2/22/2024)
Chorzempa, M. and Sacks, S. China's new rules on data flows could signal a shift away from security toward growth. Peterson Institute for International Economics. October 3, 2023.
Memorandum: Ensuring Free, Immediate, and Equitable Access to Federally Funded Research. The White House Office of Science and Technology Policy (OSTP). August 25, 2022.
Wilkinson, M., Dumontier, M., Aalbersberg, I. et al. The FAIR Guiding Principles for scientific data management and stewardship. Sci Data, 2016, 3. https://doi.org/10.1038/sdata.2016.18
Alharbi, E., Skeva, R., Juty, N., et al. Exploring the Current Practices, Costs and Benefits of FAIR Implementation in Pharmaceutical Research and Development: A Qualitative Interview Study. Data Intelligence 2021; 3 (4): 507–527. doi: https://doi.org/10.1162/dint_a_00109
Cost-benefit analysis for FAIR research data. Publications Office of the European Union. PwC EU Services. 2018. (accessed 02/22/2024).