Introduction: Turning Raw Data into Actionable Insights
Data is an invaluable resource in research. It’s essential to optimizing R&D, adopting AI, and overcoming the innovation and financial burdens that have been plaguing many R&D industries for years. But as many scientists will attest, data can also be their biggest headache. These experts know firsthand the struggle to manage and gain insights from an exploding volume of scientific data trapped in different systems and formats. They know the hassle of moving, formatting, collating, and mapping data; preparing data for analysis; correlating results and searching for the bigger picture.
For many teams, haphazard data architectures and inefficient processes are hindering researchers’ efficiency and innovation. These data struggles will become even more glaring as teams turn to AI models, which need multimodal data that is trustworthy, well formatted, well correlated, and machine ready. Data is the essential precursor to using AI to guide innovation and optimize R&D; but, many teams are struggling to find a better way to smartly collect their R&D data, break down silos, and reduce the burden of data aggregation and preparation; they need to find a faster route to get from raw data to the advanced data analyses that help accelerate life-changing discoveries. Dotmatics is here to help.
Dotmatics Luma: Complete Data-Analytics Lifecycle Support
Dotmatics Luma™ is a brand new scientific data platform that lets R&D teams integrate and interpret data from every source so they can get from instrument to insight faster.
Luma provides complete data-analytics lifecycle support from ingestion to processing to analysis.
1. Ingest Data
In today’s multimodal R&D environment, data is being generated by a wide range of lab-data producers, including instruments, registries, scientific applications, ELNs, libraries, files, etc. This huge volume of diverse data typically exists in various encrypted and vendor-specific outputs. As a result, it must be collected and annotated before it can actually be collated and used. This time-consuming and error-risking obstacle steals away time that could be better spent analyzing and applying incoming R&D data.
Dotmatics Luma Difference: Complete Instrument Integration
Dotmatics Luma uses its connected-lab solution, Luma Lab Connect, to upload data directly from virtually any equipment from any manufacturer. Files can come from a variety of sources, including Windows, Linux, S3, or API. Luma can integrate with these diverse data sources to pull in data of varying volume, type, or format, including structured, semi-structured, unstructured, sequence, numeric, text, image, metadata, etc. To support both the variety and volume of instruments in the lab, Luma currently offers more than 50 out-of-the-box parsers, providing support for hundreds of different instrument types. In terms of scale, the system can seamlessly handle file uploading from more than 1500 physical instruments. Luma also provides tools to remotely manage connected agents, getting teams up and running quickly and simplifying any configuration changes needed down the line.
2. Prepare Data
Because acquired raw data is typically unstructured, researchers cannot really do much with it until it is processed for use. Teams need to take raw data, which exists in different formats from different tools, and somehow structure and correlate it. For many teams, this involves an inordinate amount of wrangling data and writing code in order to properly collate, enrich, structure, normalize, and correlate different data sets so they can be modeled, visualized, and used for decision support. In many cases, researchers are at the mercy of data scientists or lab IT support, whose help is needed to curate and prepare requested datasets.
Dotmatics Luma Difference: Centralized Cloud Processing
Dotmatics Luma centralizes and standardizes diverse multimodal R&D data on a common, cloud-based data platform. Built using robust technologies, including AWS, Databricks, and Datadog, Luma can process diverse data at scale and ready it for downstream use. Teams can leverage out-of-the-box data models, or build their own, to transform inaccessible manufacturer data formats into usable, structured representations. With Luma, users themselves get easy-to-use tools for gathering and preparing the data sets they need to push their research forward, without relying on data scientists or lab IT for help. To provide contextualization, Luma can augment and correlate test results from instruments with experimental metadata from ELNs, assay results, endpoint data, and other key data from different Dotmatics scientific specialty tools, including those for protein characterization, flow cytometry, bioinformatics, etc. Luma also helps prepare data for meta-analysis and use within AI and ML-based algorithms by helping users collect, standardize, tag, and harmonize diverse multimodal data.
3. Analyze Data
To move from data to decision making, teams need to perform advanced analyses, visualize results, and make connections and correlations across datasets and overtime. In a multimodal R&D environment, different experts rely on different specialty software to do such—a fact that can keep teams working in isolation, with their data and analyses siloed. For many teams, their different specialty applications don’t feed into a united cloud platform, where common data models can help standardize, harmonize, and correlate diverse data and ready it for advanced analysis, including AI and ML modeling that relies on well structured, contextualized, and correlated data.
Dotmatics Luma Difference: Self-Service Data Access and Advanced Analytics
Luma empowers users to find, share, and output harmonized data into workflows, specialty apps, analytics and modeling programs, and AI/ML algorithms that help them garner insights and optimize R&D efforts. With Luma, R&D teams can:
Find data faster than ever. Luma helps teams to quickly gather and relate complex data sets for analysis thanks to its ability to create and execute queries using natural language and GenerativeAI.
Analyze data with scientific tools that researchers know and trust. Luma enables advanced scientific analysis workflows by connecting directly to the industry-leading applications, such as OMIQ, Geneious, and Prism. Users can also choose to integrate with third-party tools or build custom applications with Luma’s low-code app building tools.
Visualize and make sense of complex datasets. Luma will provide out-of-the-box dashboards and visualizations to help users scrutinize data and accelerate decision making, or it can link to third-party visualization tools.
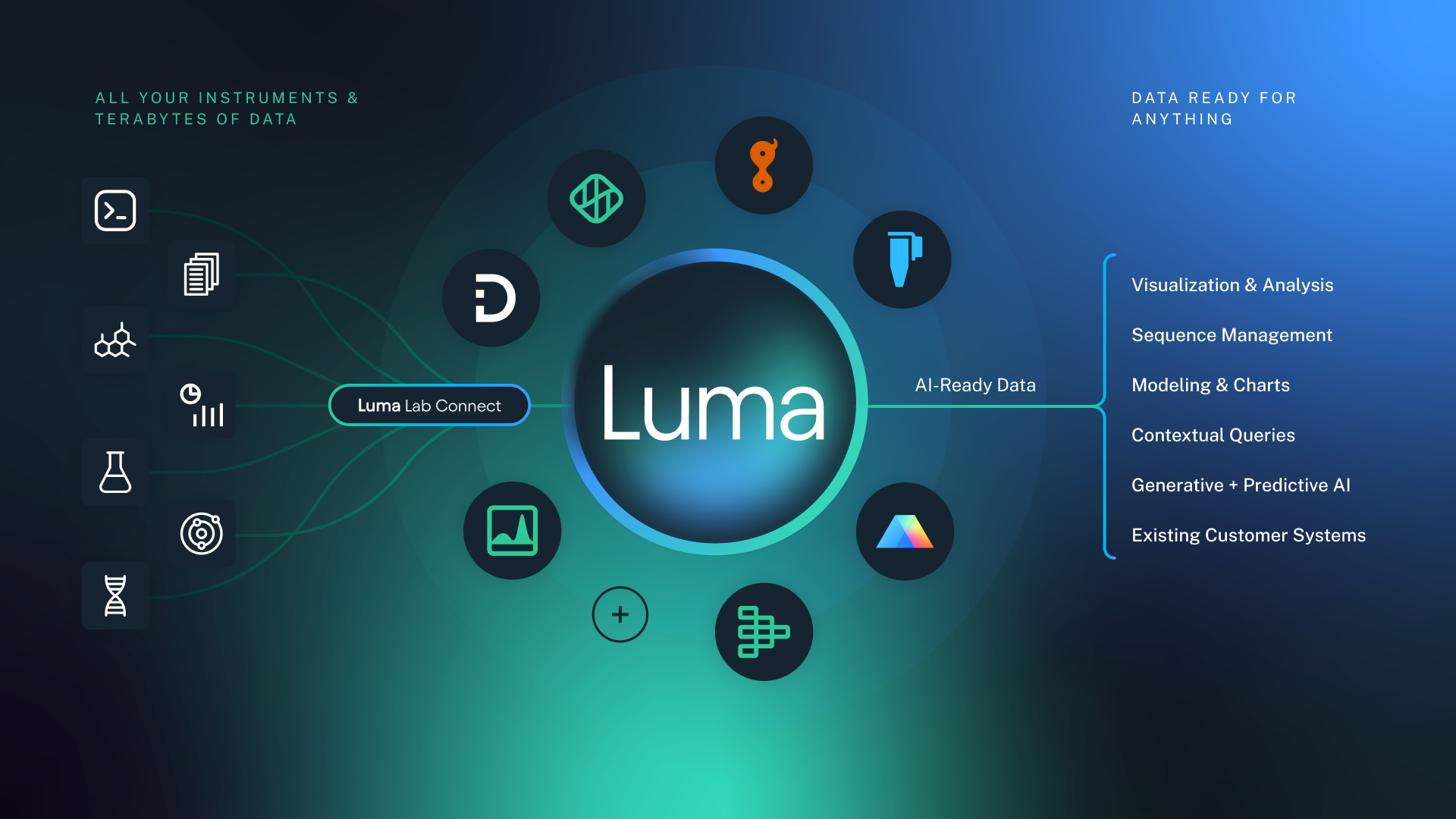
Dotmatics Luma manages the full data-analytics lifecycle on one platform. Left: Ingest multimodal R&D data from any disparate source. Center: Centralize data storage and processing to prepare diverse, unstructured data for downstream use. Right: Uncover insights by querying, modeling, and analyzing harmonized data.
Learn More about Dotmatics Luma
Want to learn more about Dotmatics Luma?